分布式适配
在单卡模式下已经成功完成迁移,我们开始分布式模式的迁移,分布式迁移并不影响单卡功能,您的分布式脚本和单卡脚本最终是同一份脚本,可以同时以单卡或分布式方式执行。我们依次按照分布式迁移的过程开始迁移。
- worker间变量初值同步
根据脚本逻辑找到模型创建完成的位置official/vision/image_classification/resnet/resnet_ctl_imagenet_main.py,添加可训练变量的同步操作,这里需要使用npu.distribute.broadcast接口。
1 2 3 4 5
with distribute_utils.get_strategy_scope(strategy): # 模型创建 runnable = resnet_runnable.ResnetRunnable(flags_obj, time_callback, per_epoch_steps) # 变量同步 npu.distribute.broadcast(runnable.model.trainable_variables)
- worker间梯度聚合
根据脚本逻辑,我们找到训练过程中梯度更新的部分official/vision/image_classification/resnet/resnet_runnable.py
1 2 3 4 5 6 7 8 9 10 11 12 13 14 15 16 17 18 19 20 21 22 23 24 25 26 27 28 29 30
def train_step(self, iterator): """See base class.""" def step_fn(inputs): """Function to run on the device.""" images, labels = inputs with tf.GradientTape() as tape: logits = self.model(images, training=True) prediction_loss = tf.keras.losses.sparse_categorical_crossentropy( labels, logits) loss = tf.reduce_sum(prediction_loss) * (1.0 / self.flags_obj.batch_size) num_replicas = self.strategy.num_replicas_in_sync l2_weight_decay = 1e-4 if self.flags_obj.single_l2_loss_op: l2_loss = l2_weight_decay * 2 * tf.add_n([ tf.nn.l2_loss(v) for v in self.model.trainable_variables if 'bn' not in v.name ]) loss += (l2_loss / num_replicas) else: loss += (tf.reduce_sum(self.model.losses) / num_replicas) grad_utils.minimize_using_explicit_allreduce( tape, self.optimizer, loss, self.model.trainable_variables) self.train_loss.update_state(loss) self.train_accuracy.update_state(labels, logits)
这里可以看出,TF2原始脚本中,使用了函数minimize_using_explicit_allreduce来屏蔽部署形态,进入函数内部,可以找到实际执行梯度聚合的函数在:official/staging/training/grad_utils.py。
1 2 3
def _filter_and_allreduce_gradients(grads_and_vars, allreduce_precision="float32", bytes_per_pack=0):
这里需要注意,我们要求以单卡CPU的形式启动训练,所以,此时代码中的原始梯度聚合行为不生效(或者说因为是单卡,所以不需要聚合),在这个函数内部,我们需要添加NPU上的梯度聚合操作,这里需要用到npu.distribute.all_reduce接口。我们在official/staging/training/grad_utils.py添加如下信息:
1 2 3 4 5 6 7 8 9 10 11 12 13 14 15 16 17 18
# 由于需要使用npu.distribue.all_reduce接口,在脚本开头import npu import npu_device as npu def _filter_and_allreduce_gradients(grads_and_vars, allreduce_precision="float32", bytes_per_pack=0): ... ... # 原始脚本采用SUM策略 allreduced_grads = tf.distribute.get_strategy( # pylint: disable=protected-access ).extended._replica_ctx_all_reduce(tf.distribute.ReduceOp.SUM, grads, hints) if allreduce_precision == "float16": allreduced_grads = [tf.cast(grad, "float32") for grad in allreduced_grads] # 由于NPU适配添加的梯度聚合操作,聚合类型保持与原始脚本一致,此处选择“sum”聚合策略 allreduced_grads = npu.distribute.all_reduce(allreduced_grads,reduction="sum") return allreduced_grads, variables
- 不同worker上的数据集分片
根据脚本逻辑找到预处理函数official/vision/image_classification/resnet/resnet_runnable.py:
1 2 3 4 5 6 7 8 9 10 11 12
# 假数据,忽略该分支 if self.flags_obj.use_synthetic_data: self.input_fn = common.get_synth_input_fn( height=imagenet_preprocessing.DEFAULT_IMAGE_SIZE, width=imagenet_preprocessing.DEFAULT_IMAGE_SIZE, num_channels=imagenet_preprocessing.NUM_CHANNELS, num_classes=imagenet_preprocessing.NUM_CLASSES, dtype=self.dtype, drop_remainder=True) else: # 真实的预处理方法 self.input_fn = imagenet_preprocessing.input_fn
找到official/vision/image_classification/resnet/imagenet_preprocessing.py,添加如下信息:
1 2 3 4 5 6 7 8 9 10 11
# 由于需要使用npu.distribue.shard_and_rebatch接口,在脚本开头import npu import npu_device as npu if input_context: logging.info( 'Sharding the dataset: input_pipeline_id=%d num_input_pipelines=%d', input_context.input_pipeline_id, input_context.num_input_pipelines) # 原始的shard逻辑,因为以单机CPU方式启动,所以不会进行实际的shard dataset = dataset.shard(input_context.num_input_pipelines, input_context.input_pipeline_id) # NPU添加的shard逻辑,会根据集群数量,对数据集和全局batch进行切分 dataset, batch_size = npu.distribute.shard_and_rebatch_dataset(dataset, batch_size)
执行完上述步骤后,分布式迁移完成。
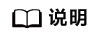
新增的调用对单卡流程没有任何影响,这些接口内部会根据是否设置了NPU分布式执行的环境变量来决定是否生效。如果是单卡模式执行,这些接口不会执行任何操作。